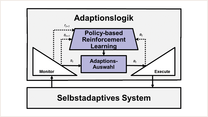
The Port of Duisburg is the largest inland container port in the world; it handles more than 4 million containers each year. With the increasing digitalization of the terminals, larger volumes of precise data about terminal processes are being created. The gantry cranes alone, which move containers between trains and trucks, produce data for around 100 variables at five-second intervals (including the crane’s position, energy consumption, error states, etc.).
This data can be used for predictions, for example, about whether a train will leave the terminal on time. This enables terminal operators to proactively address impending delays by, for example, increasing staffing levels. However, as we know from the weather, predictions are more accurate the shorter the forecast period is. At the same time, terminal operators are faced with the problem that interventions in ongoing processes need a certain lead time to become effective. The paluno working group of Prof. Pohl and the Port of Duisburg wanted to know how they could support terminal managers in this tradeoff between accurate predictions and early intervention.
Ensemble Deep Learning Delivers Precise Predictions
To this end, the research team developed a prototype for a “Terminal Productivity Cockpit”. In addition to predictions themselves, this system provides reliability estimates for the predictions. The calculation is based on combining up to one hundred prediction models into an ensemble. The individual prediction models are first trained based on historical process data by means of deep machine learning. The Ensemble Deep Learning has two advantages: on the one hand, the predictions of an ensemble are more accurate than the predictions of individual prediction models. On the other hand, the reliability of the predictions can be calculated based on the number of matching prediction models — similar to the Ask the Audience lifeline in “Who Wants to Be a Millionaire?”.
In order to assess their approach, the researchers analyzed potential improvements in terminal operations in terms of productivity and costs. The punctuality of the outgoing trains served as an indicator for productivity. According to the analysis, the increase in the productivity of the terminal with machine-based decision support was estimated to be approximately 5%.
New Platform to Ensure Data Quality
However, in order for ports to actually benefit from such modern methods of data analysis and machine learning, the data must be properly integrated and processed. In the “Terminal Productivity Cockpit” prototype, these steps represented approximately 80% of development effort. To make the process more efficient, together with European partner, paluno researchers are working on a domain-specific data platform for ports in the DataPorts project.
Further Information
The work was conducted within the framework of the European big data lighthouse project TransformingTransport. A success story about the project has recently been published on the European Commission’s website. Overall, TransformingTransport showed how big data and artificial intelligence (AI) can transform the European transport sector by reducing transport costs and fuel consumption while increasing the efficiency of transport operators: Big data offers big gains for transport operators
In the EU Big Data Value Public-Private Partnership (PPP) project DataPorts (https://dataports-project.eu/), the working group of Professor Pohl together with international partners is developing a data platform for European seaports. The project addresses, among other things, the problem of data processing, data aggregation, and the acquisition of large volumes of data.
Publications
A. Metzger, J. Franke, and T. Jansen, “Ensemble deep learning for proactive terminal process management at the port of Duisburg ’duisport’,” in Business Process Management Cases – Volume 2, J. vom Brocke, J. Mendling, and M. Rosemann, Eds. Springer, 2020 – to be published.
A preliminary open access version is available at http://ceur-ws.org/Vol-2428/paper17.pdf (A. Metzger, J. Franke, and T. Jansen, “Data-driven deep learning for proactive terminal process management,” in Proceedings of the Industry Forum at BPM 2019 co-located with 17th International Conference on Business Process Management (BPM 2019), Vienna, Austria, September 1-6, 2019., ser. CEUR Workshop Proceedings, J. vom Brocke, J. Mendling, and M. Rosemann, Eds., vol. 2428. CEUR-WS.org, 2019, pp. 190–201)
Contact
Software Systems Engineering (SSE) | +49 201 18-34650 andreas.metzger@paluno.uni-due.de |
Press and Public Relations | +49 201 18-34655 birgit.kremer@paluno.uni-due.de |